
We show here that inhibitory recurrent dynamics and sparse distributed representations make our model more robust in the face of ambiguity, by testing recognition performance with occluded visual inputs. The sparse distributed representations produced by these recurrent inhibitory dynamics have been shown to produce biologically realistic representations in response to natural stimuli (e.g., O’Reilly and Munakata, 2000 Olshausen and Field, 2004 O’Reilly et al., 2012). We demonstrate how recurrent excitatory processing could provide a similar function in visual occlusion, which requires the organization of image fragments that span multiple receptive fields into a logical whole Gestalt and involves the filling-in of missing visual information ( Kourtzi and Kanwisher, 2001 Lerner et al., 2002 Rauschenberger et al., 2006 Weigelt et al., 2007 Wyatte et al., 2012a).Īt a more local level, recurrent inhibitory processing produces sparse distributed representations, implemented in LVis through the use of a k-Winners-Take-All (kWTA) inhibition function (where k represents the roughly 15–25% activity levels present in neocortical networks O’Reilly, 1998 O’Reilly and Munakata, 2000 O’Reilly et al., 2012). Recurrent processing, for example, has been suggested to be critical for solving certain visual tasks such as figure-ground segmentation ( Hupe et al., 1998 Roelfsema et al., 2002 Lamme and Roelfsema, 2000), which requires integration of information from outside the classical receptive field. We describe an explicit computational model ( LVis – Leabra Vision) of the function of this recurrent architecture in the context of visual object recognition, demonstrating a synergy between the learning and processing benefits of recurrent connectivity. There are extensive bidirectional excitatory and inhibitory connections within individual cortical areas, and almost invariably, every area that receives afferent synapses from another area, also sends back efferent synapses in return ( Felleman and Van Essen, 1991 Scannell et al., 1995 Sporns and Zwi, 2004 Sporns et al., 2007). One of the most salient features of the mammalian neocortex is the structure of its connectivity, which provides for many forms of recurrent processing, where neurons mutually influence each other through direct, bidirectional interactions. Altogether, these findings support the potential importance of ongoing recurrent processing throughout the brain’s visual system and suggest ways in which object recognition can be understood in terms of interactions within and between processes over time. Consistent with this prediction, we show how semantic knowledge about object categories changes the nature of their learned visual representations, as well as how this representational shift supports the mapping between perceptual and conceptual knowledge. Furthermore, this interaction of recurrent connectivity and learning predicts that high-level visual representations should be shaped by error signals from nearby, associated brain areas over the course of visual learning. Both the unified, biologically plausible learning mechanism and the robustness to occlusion derive from the role that recurrent connectivity and recurrent processing mechanisms play in the model.

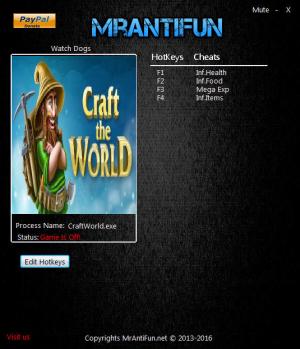
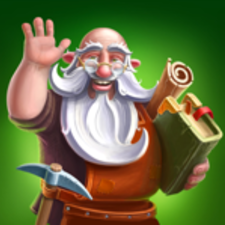
The model exhibits robustness to highly ambiguous, partially occluded inputs.

How does the brain learn to recognize objects visually, and perform this difficult feat robustly in the face of many sources of ambiguity and variability? We present a computational model based on the biology of the relevant visual pathways that learns to reliably recognize 100 different object categories in the face of naturally occurring variability in location, rotation, size, and lighting. 1Department of Psychology and Neuroscience, University of Colorado Boulder, Boulder, CO, USA.O’Reilly 1,2* †, Dean Wyatte 1* †, Seth Herd 1,
